How can machine learning be both fair and accurate?
- Soujanya Syamal
- Oct 25, 2021
- 2 min read
When it comes to utilising machine learning to make public policy decisions, researchers at Carnegie Mellon University are questioning a long-held belief that there is a trade-off between accuracy and fairness.

Concerns have grown as the use of machine learning has grown in areas such as criminal justice, hiring, health care delivery, and social service interventions, raising questions about whether such applications introduce new or amplify existing inequities, particularly among racial minorities and people with low income. Adjustments are made to the data, labels, model training, scoring systems, and other parts of the machine learning system to defend against this bias. The underlying theoretical assumption is that the system will become less accurate as a result of these modifications.
In new research just published in Nature Machine Intelligence, a CMU team hopes to refute that belief. Rayid Ghani, a professor in the School of Computer Science's Machine Learning Department and the Heinz College of Information Systems and Public Policy; Kit Rodolfa, a research scientist in ML; and Hemank Lamba, a post-doctoral researcher in SCS, tested that assumption in real-world applications and discovered that the trade-off was negligible in practice across a range of policy domains.
"You can truly obtain both. You don't have to forgo precision to create fair and equal processes "Ghani said. "However, it does need the conscious creation of fair and equitable processes. Off-the-shelf solutions aren't going to cut it."
Ghani and Rodolfa concentrated on circumstances in which in-demand resources are restricted and machine learning techniques are utilised to assist in resource allocation. The researchers looked at four systems: prioritising limited mental health care outreach based on a person's risk of returning to jail to reduce reincarceration; predicting serious safety violations to better deploy a city's limited housing inspectors; modelling the risk of students not graduating from high school on time to identify those who need additional support; and assisting teachers in reaching crowdfunding goals for classroom needs.
In each case, the researchers discovered that models tuned for accuracy—a common strategy in machine learning—could accurately predict the desired results, but there were significant differences in intervention recommendations. When the researchers made tweaks to the models' outputs aimed at increasing fairness, they observed that discrepancies based on race, age, or income—depending on the situation—could be addressed without sacrificing accuracy.
Ghani and Rodolfa believe that their findings will persuade other researchers and policymakers to reconsider using machine learning in decision-making.
"We urge the artificial intelligence, computer science, and machine learning groups to stop assuming that accuracy and justice are mutually exclusive and instead start creating systems that optimise both," Rodolfa said. "We expect that policymakers will use machine learning as a decision-making tool to assist them to attain more egalitarian outcomes."
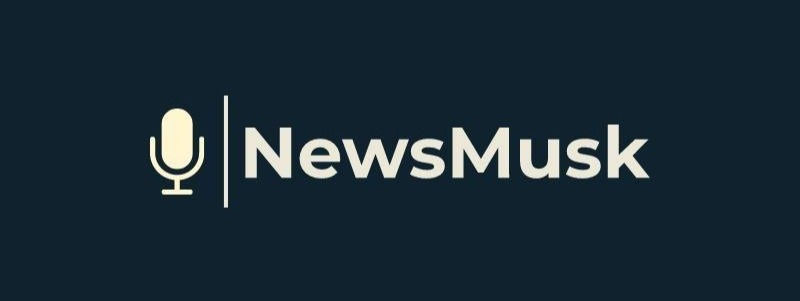
reference- techexplore
In the endless runner game Temple Run 2, you take control of an explorer escaping a terrifying beast in a temple that has been cursed.
Prepare for your Power BI certification exam with confidence at WebAsha Technologies in Pune. Our Power BI Exam Preparation program is tailored to help you master the concepts and skills needed to excel in the certification tests. With a focus on practice sessions, mock exams, and personalized guidance, we ensure you’re fully equipped to achieve success.
If you love challenges and creativity in music, Sprunki Incredibox is the perfect place for you to show off your talent. Try out amazing sounds, and blend them to create the music you've always dreamed of. You'll feel excited every time you play.
https://ftgam.online/
https://gamshf.online/
https://reapk.xyz/
https://ebilos.com/
https://karachistartups.com/
https://clgames.xyz/
https://gamsun.xyz/
Jobs in Umm Al Quwain
UAE multiple vacancies
Jobs in Sharjah
Jobs in Fujairah
Jobs in Qatar
Waiter Jobs
Airport jobs